Climate Futures has several partners, one of them being the Norwegian School of Economics (NHH). In the spring of 2021, the leader of the Smart Shipping node, Roar Ådland, supervised a master thesis that investigated the seasonality in weather dependent shipping fuel costs titled “Estimating weather margin seasonality in shipping using machine learning”. Shipping companies can minimize their financial risk by knowing the weather conditions for a route well in advance, and seasonal forecasts can be a useful tool for this. This article will sum up some of the main points of the thesis and elaborate a bit on how Climate Futures works in the Smart Shipping node.
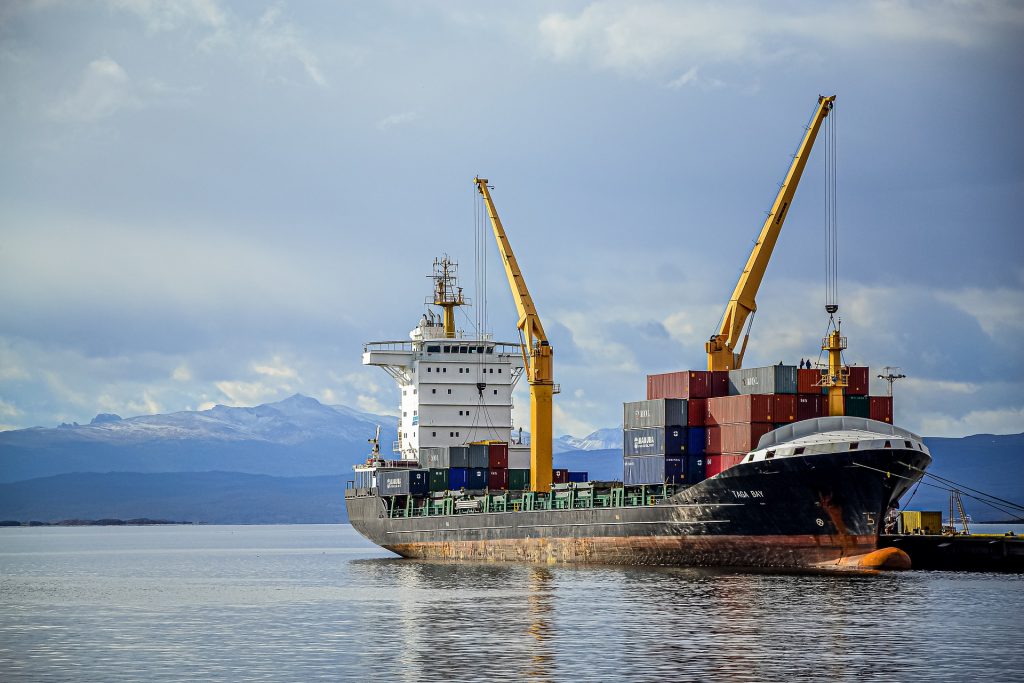
Why estimate weather conditions in the shipping industry?
The main reason for estimating weather conditions in the shipping industry is to get better pricing and less financial risk. This fits well with one of the aims of the shipping node, which is to measure the costs of operating ships and to try to optimise how ships are operated, for example through the choice of speed and route to reduce emissions.
It might seem obvious that consumption of fuel is bigger in the North Atlantic Ocean during winter than in the South-East Asian water on a calm summer day, but vessel operators fail to be able to quantify this difference to better estimate how much fuel the ships actually use. A combination of uncertainty in weather prediction models and high variance in weather conditions makes it difficult to accurately predict fuel consumption, even for voyages not so long into the future. The longer into the future you plan for, the harder it becomes to predict what weather conditions you will encounter during a voyage.
So how can we predict fuel consumption when we are planning a voyage several weeks, even months, into the future? Making predictions without knowing the weather conditions is harder than it may seem. One approach is to look at historical data – average consumption or average weather conditions along a route at a given time of year and use this to generate an estimate. To further improve the estimates, one must also account for the complex relationship between many variables, such as cargo weight and ship speed, most of which are not known in advance.
Why use seasonal forecasting in the shipping industry?
To create fairly accurate fuel consumption is not necessarily that difficult in itself but knowing the weather conditions is the tricky part. We normally have access to forecasts, but they do not predict the weather far enough into the future. This is where seasonal forecasts come in.
In this thesis, machine learning tools were created that made predictions within 2-3% accuracy for a given voyage. However, these can only be used if the weather conditions are known. Since voyages are usually planned several weeks in advance, short term forecasts cannot directly be used to make predictions.
The variation in prediction accuracy highlights the need for seasonal forecasting: when weather conditions are known, the best models can predict consumption for a voyage with low single-digit percentage errors. Without knowing what the weather will be like, we are left with broad consumption estimates that are quite inaccurate. Seasonal forecasting is an important tool in bridging the gap in accuracy and help reduce uncertainty.
However, there are also many other reasons why seasonal and sub-seasonal weather forecasting can be extremely important in shipping. Firstly, we know that weather directly affects the demand for shipping. For instance, extreme cold or hot weather directly affects energy demand, and implicitly the demand for the global transportation of gas and other energy sources. Precipitation patterns also affect the harvests of grain and other agricultural commodities and may reduce the exports of these by wreaking havoc on infrastructure through the flooding of mines and railways or affecting the water level for river barges. Having a better handle on such risks can help shipping companies to better position their fleet globally, both to improve financial performance but also to reduce the physical risk for their fleet.
About the thesis
The approach outlined in this thesis relies on publicly available historical weather data together with more traditional machine learning models for estimating fuel consumption. This approach makes the consumption models more generalizable and easier to compare with other models. Moreover, seasonal forecasts are relevant for use by shipping operators since they can generate good estimates further into the future. All in all, by having access to better models and knowing the fuel consumption, the efficiency and therefore also sustainability in the shipping industry can be improved.
There has been a lot of previous research on developing good fuel prediction models, and a few even used third-party weather data, just like this thesis did. Nevertheless, what is new in this thesis is that the authors used trained models that consider the relation between weather and fuel consumption together with historical weather data to predict consumption given the historical weather conditions along the route. In doing so, they were able to use machine learning models to make predictions without knowing the weather conditions. Relying on historical weather patterns instead, they could calculate expected consumption and consumption variance for a given voyage.
The framework presented in this thesis can indicate the cost and risk associated with a route and in turn improve the pricing of cargo. This research is a good example of how Climate Futures can take raw data and turn it into something useful, applicable to the real world.
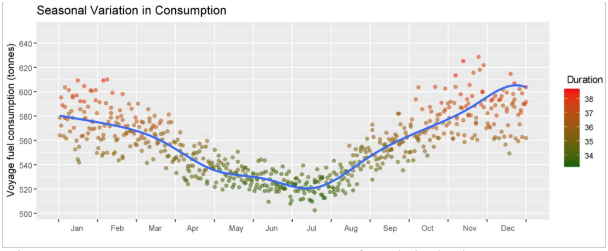
As illustrated by the figure above, the models used in the thesis indicate that not only does fuel consumption increase during winter because of more volatile weather conditions, thus consuming more fuel per day, but additionally, a voyage typically lasts for several days which also increases the total fuel consumption compared to summer.
These results show that accurate weather forecasts are useful to get a better estimate of fuel consumption during winter voyages. The term weather margins in this thesis refers to the “increase in consumption due to weather compared to consumption in ideal conditions.” (Nilsson & Nilsson, 2021). Using historical weather conditions to generate predictions, the thesis found that weather margins deviate more than twice as much from average during winter than summer. This poses a big financial risk.
Today, the dry bulk freight market is much stronger financially than it has been the last several years. Consequently, relatively small fuel savings are less important than increasing revenue through higher ship utilization. Dry bulk operators will therefore risk using more money than necessary on fuel if it means that more of their vessels are in operation. However, as markets are cyclical, there will come a time when ship owners and operators again focus on the smaller costs and estimating fuel consumption will be prioritised.
In conclusion, more accurate weather forecasts can result in better estimates of weather margins that lead to a more accurate estimate of fuel consumption. This in turn will potentially give a better indication of cost and risk associated with a certain route, which can be used to improve the pricing of cargo in the shipping industry.
How will the shipping node continue to work on these issues?
As an extension of this thesis’ work, the shipping node is planning to investigate two related practical applications. Firstly, we will try to model how increasing sea temperatures affect marine growth on ships’ hulls, thus contributing to higher fuel consumption. Secondly, we want to consider the impact of seasonal variations in river water levels in South America on the demand for ships and the related pricing of cargoes for operators, such as our partner Western Bulk. Much of this work will again be performed as part of future master thesis work at NHH.
Finally, it is worth mentioning that estimating fuel consumption based on historical data can be problematic in a rapidly changing climate. The next 20 years will in all likelihood be increasingly affected by extreme weather than the past 20 years have been, so estimating weather conditions based on previous years can give inadequate results. An extension of this work could be to project how increasingly volatile weather conditions in the future will increase the cost of international ocean transportation. This is something we have thought about and might want to do within Climate Futures.
Sources/Further read:
Nilsson, J. & Nilsson, M. (2021). Estimating weather margin seasonality in shipping using machine learning. Master’s thesis, NHH.