One of our projects, Seasonal Forecasting Engine, recently published their June forecast based on five numerical weather prediction models. The June forecast was remarkable in that it predicted almost no temperature anomalies in Northern Europe. When we sum up in July, we will probably find that some regions experienced either warm or cold anomalies, but there was no consistent signal across the models this time.
In Climate Futures, we will make use of advanced statistics and machine learning to create algorithms that improve the skill of forecasts going from 10 days to 10 years into the future. The key will be to learn how different climate variables interact and then to use that knowledge to make better use of the physical models. Erik Kolstad, the PI of the SFE project, was the lead author of a 2017 article on the different roles that snow cover, soil moisture and soil temperature play in dictating the persistence of temperature from month to month.
A key figure in that paper showed which of these variable were the most important mediator of persistence in winter and summer:
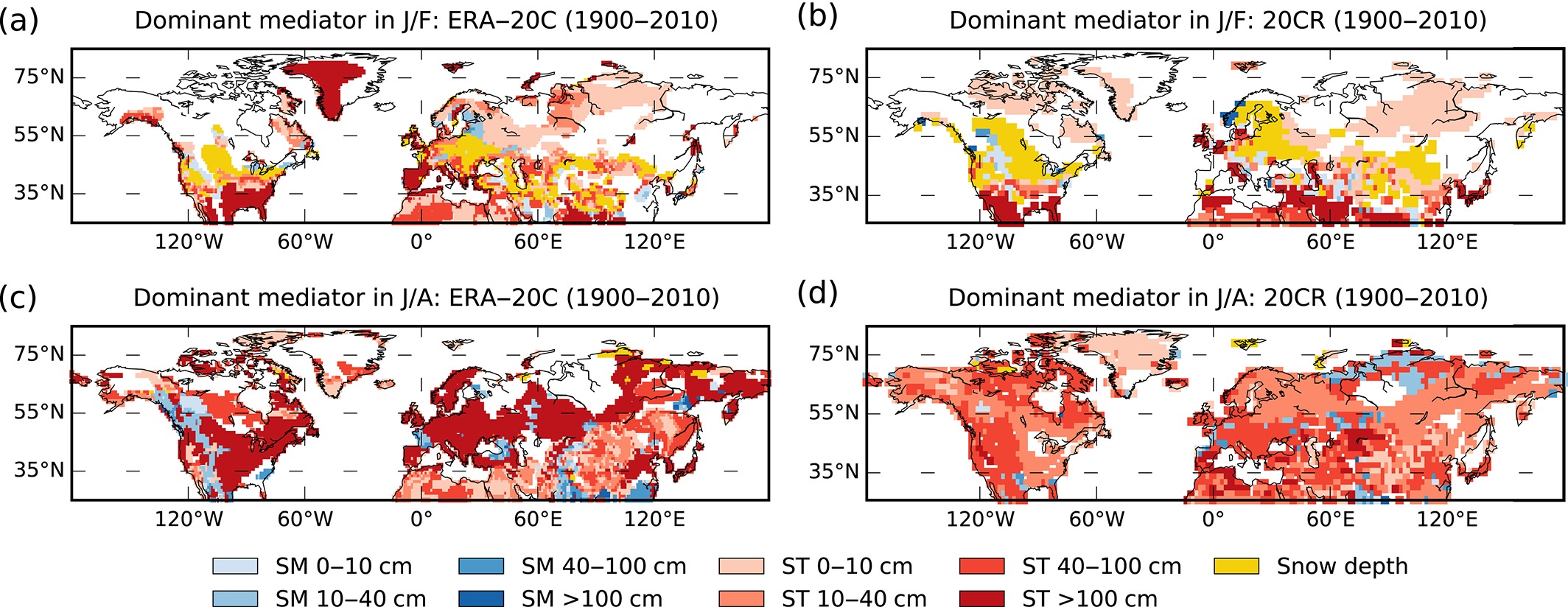
At midlatitudes, snow depth plays an important role. This means that when there is a lot of snow on the ground, the temperature will probably stay anomalously cold from January to February. Or if there is thin snow or no snow at all, the temperature will be anomalously warm in both months. The specific role of snow was examined in a follow-up study. In summer, soil variables dominate completely.
Our long-term goal is to assign a higher weight to the models that represent these physical mechanisms well while assigning a lower weight to less realistic models. To be able to plough through the huge data amounts available from models, observations and satellite imagery, we need to let computers do the heavy lifting. Another important reason not to do this manually is that humans are prone to making mistakes, often in the form of being too subjective. Machines, for better or worse, do not have this bias, which we believe makes them ideally suited for this work.
Stay tuned for our July forecast and other developments!